UOMOP
미니배치 경사 하강법 using algorithm (boston) 본문
미니배치 경사 하강법은 샘플의 일부만을 이용해서 선형식의 가중치와 절편을 업데이트하고, 샘플의 양은 설정이 가능.
미니배치 경사 하강법을 크게 2가지 방식이 있다.
학습 샘플을 특정 갯수만큼 랜덤하게 추출하거나,
학습 샘플을 특정 갯수만큼 가져오되, 모든 데이터를 사용할 수 있도록 하는 것이다.
실무에서는 2번째 방식을 더 많이 이용하여 데이터 편향을 방지하여 모든 데이터를 학습할 수 있도록 한다.
실제로 keras에서도 2번째 Mini-Batch Gradient Descent 방식을 자동으로 사용하고 있고, batch_size의 default값은 32이다.
1. 데이터 불러오기
import numpy as np
import pandas as pd
from sklearn.datasets import load_boston
boston = load_boston()
boston_df = pd.DataFrame(boston.data, columns = boston["feature_names"])
boston_df["PRICE"] = boston.target
2. mini_Batch Gradient Descent 함수 정의
def mini(feature, target, learning_rate, iter_epochs, batch_size, verbose):
bias = np.random.rand(1,)
w1 = np.random.rand(1,)
w2 = np.random.rand(1,)
print("####### 최초 bias, w1, w2 #######\n")
print("bias : {}\tw1 : {}\tw2 : {}\n\n\n".format(bias, w1, w2))
for i in range(iter_epochs) :
for batch_step in range(0, len(target), batch_size):
feature_1 = feature[batch_step : batch_step + batch_size, 0]
feature_2 = feature[batch_step : batch_step + batch_size, 1]
target_in = target[batch_step : batch_step + batch_size]
N = len(target_in)
predict = bias + w1 * feature_1 + w2 * feature_2
diff = target_in - predict
bias_update = -(2/N) * learning_rate * (np.dot(np.ones((N,)), diff))
w1_update = -(2/N) * learning_rate * (np.dot(feature_1.T, diff))
w2_update = -(2/N) * learning_rate * (np.dot(feature_2.T, diff))
##########################################################################
predict_for_loss = bias + w1 * feature[:, 0] + w2 * feature[:, 1]
diff_for_loss = target - predict_for_loss
err = np.mean(np.square(diff_for_loss))
#########################################################################
bias = bias - bias_update
w1 = w1 - w1_update
w2 = w2 - w2_update
if verbose == True :
print("Epoch( {}/{})\tbatch_step = {}".format(i+1, iter_epochs, batch_step))
print("bias : {}\tw1 : {}\tw2 : {}\terror : {}\n".format(bias, w1, w2, err))
return bias, w1, w2
3. feature 전처리(Scaling)
from sklearn.preprocessing import MinMaxScaler
mms = MinMaxScaler()
scaled_feature = mms.fit_transform(boston_df[["RM", "LSTAT"]])
4. GD를 통해 가중치, 절편 확인
bias, w1, w2 = mini(scaled_feature, boston_df["PRICE"], learning_rate = 0.01,
iter_epochs = 2000, batch_size = 30, verbose = True)
5. 도출해낸 가중치, 절편으로 선형식을 완성하고, 예측 진행
z = bias + w1 * scaled_feature[:, 0] + w2 * scaled_feature[:, 1]
print(z)
6. 예측 결과를 DataFrame에 추가
boston_df["PREDICT_using_hand"] = z
boston_df.head(5)
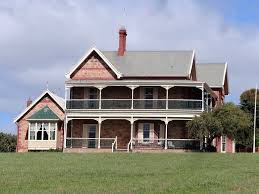
'Ai > DL' 카테고리의 다른 글
CNN model 생성 (0) | 2022.02.06 |
---|---|
Functional API (0) | 2022.02.06 |
확률적 경사 하강법 using algorithm (boston) (0) | 2022.02.01 |
배치 경사 하강법 using keras (boston) (0) | 2022.02.01 |
배치 경사 하강법 using algorithm (boston) (0) | 2022.02.01 |