목록2024/08 (20)
UOMOP
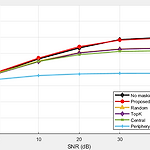
% SNRdB 값 설정snrdb = [0, 10, 20, 30, 40];% PSNR 값 데이터 초기화data.NoMasking = struct('x1024', [19.958 25.263 28.937 31.97 33.79], ... 'x512', [19.051 23.277 26.696 29.33 29.956], ... 'x256', [17.897 21.718 24.645 26.202 26.54]);data.CBM = struct('x1024', struct('x0_23', [20.571 24.169 28.232 31.348 33.606], ... 'x0_43', [20..
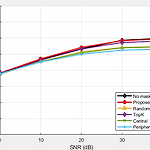
% SNRdB 값 설정snrdb = [0, 10, 20, 30, 40];% PSNR 값 데이터 초기화data.NoMasking = struct('x1024', [19.958 25.263 28.937 31.97 33.79], ... 'x512', [19.051 23.277 26.696 29.33 29.956], ... 'x256', [17.897 21.718 24.645 26.202 26.54]);data.CBM = struct('x1024', struct('x0_23', [20.485 25.515 29.246 32.048 33.168], ... 'x0_43', [20..
class Decoder(nn.Module): def __init__(self, latent_dim): super(Decoder, self).__init__() self.latent_dim = latent_dim self.linear = nn.Linear(self.latent_dim, 2048) self.prelu = nn.PReLU() self.unflatten = nn.Unflatten(1, (32, 8, 8)) self.essen = nn.ConvTranspose2d(32, 16, kernel_size=5, stride=2, padding=1, output_padding=1) self.in6 = nn.Co..
import torchimport torch.nn as nnclass Encoder(nn.Module): def __init__(self, latent_dim): super(Encoder, self).__init__() self.latent_dim = latent_dim self.in1 = nn.Conv2d(3, 16, kernel_size=5, stride=1, padding=1) self.in2 = nn.Conv2d(3, 16, kernel_size=5, stride=1, padding=1) self.in3 = nn.Conv2d(3, 16, kernel_size=5, stride=1, padding=1) self.in4 ..
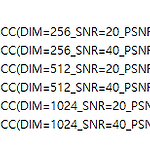
import mathimport torchimport torchvisionfrom fractions import Fractionimport numpy as npimport torch.nn as nnimport torch.optim as optimimport torch.nn.functional as fimport matplotlib.pyplot as pltimport torchvision.transforms as trfrom torchvision import datasetsfrom torch.utils.data import DataLoader, Datasetimport timeimport osfrom skimage.metrics import structural_similarity as ssimfrom pa..
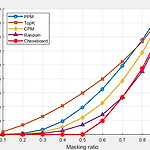
import numpy as npimport torchimport torchvisionimport torchvision.transforms as transformsdef patch_importance(image, patch_size=2, type='variance', how_many=2, noise_scale=0): if isinstance(image, torch.Tensor): image = image.numpy() H, W = image.shape[-2:] extended_patch_size = patch_size + 2 * how_many value_map = np.zeros((H // patch_size, W // patch_size)) for i in ra..